Introduction
Hot housing market? Bidding wars inflate prices. The National Association of Realtors (NAR) reports that bidding wars are happening in more than half of all home purchases today. Machine learning can help! It uses past data and powerful algorithms to guide informed bids on your dream home.
Objective
The goal is to determine the ideal bid price for a house located in a suburban town in New Jersey. This will be achieved by analyzing historical real estate data, economic indicators, and demographic data to create a predictive model.
Process
Machine learning for real estate bidding is a data-driven approach that analyzes historical data on past sales, property characteristics, and market trends. Machine learning models analyze these factors, identifying patterns and relationships that can inform your real estate bidding strategy with valuable insights. Here’s the process:
- Data Collection: We gathered 5 years of Montclair (NJ) property data (>2,000). The data comes from multiple sources, including leading real estate platforms like Zillow and Redfin.
- Data Preparation: The collected data was meticulously cleaned and aligned to ensure accuracy. Missing values were addressed, and outliers, such as properties with significantly higher or lower values than the average, were identified and managed. In addition to historical data, the model also considered relevant economic indicators and demographic information.
- Predictive Modeling: The cleaned and prepared data were used to create a predictive model. The model identified key predictors, including list price, days on market, household median income, and number of bedrooms. Finally, we used the model to predict the bid price for the target house.
Modeling Workflow: Here is a summary of the modeling workflow:
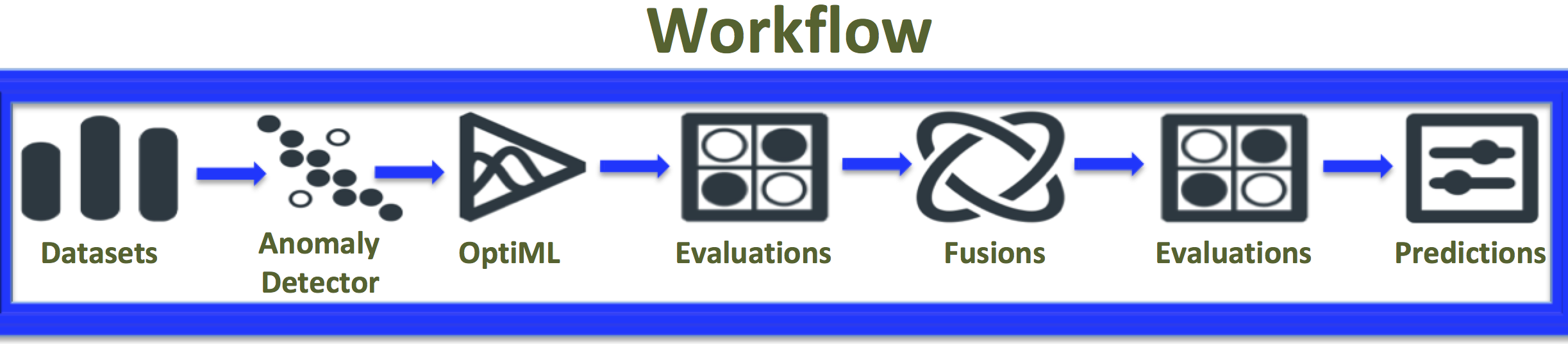
Modeling workflow
Upload the Dataset: We began by uploading the dataset containing the historical real estate, economic, and demographic data.
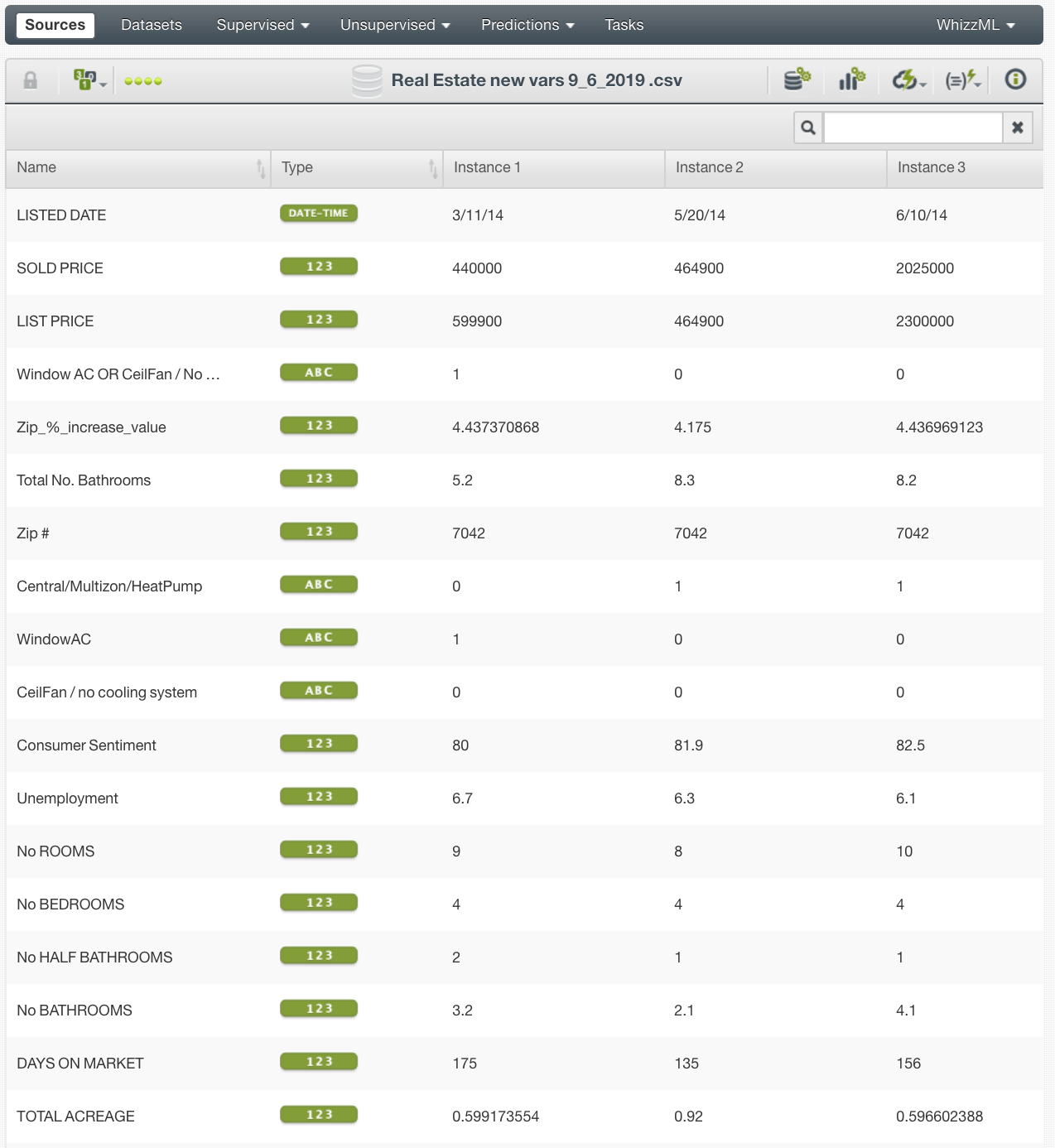
Data source
Anomaly Detection: An anomaly detector was used to clean the data by identifying and handling outliers.
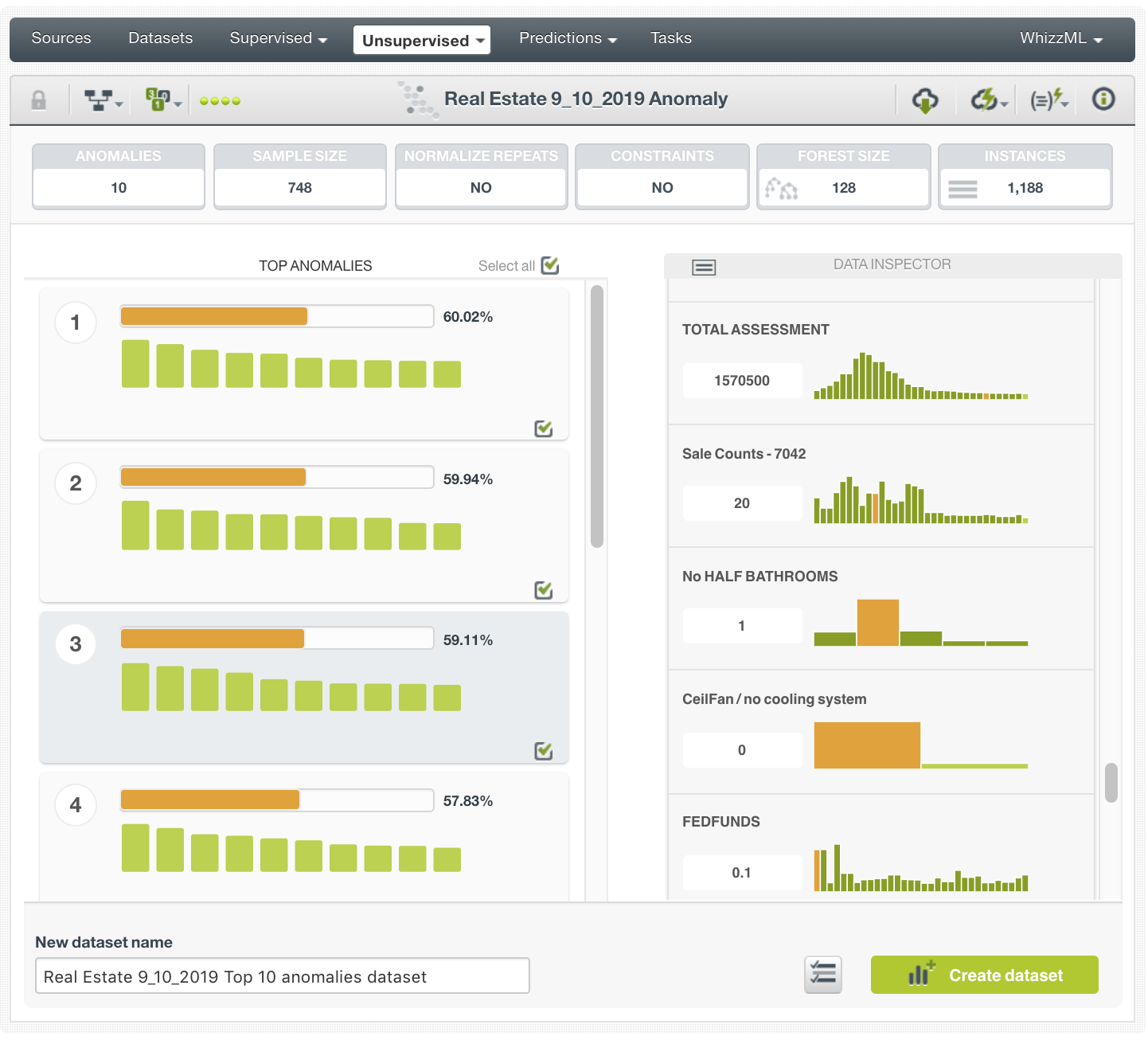
Anomaly detection
Model Training: The data was then used to train a predictive model, selecting the most influential features.
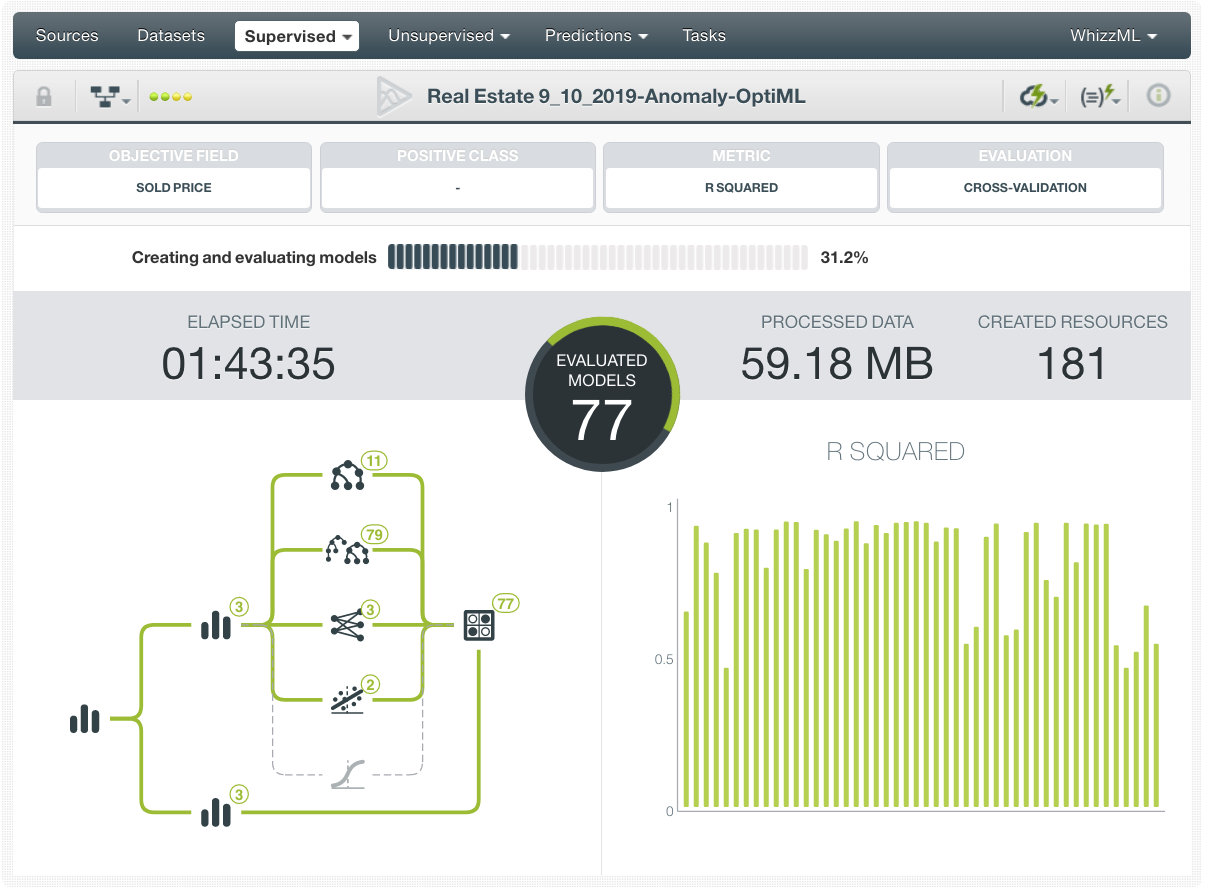
Model training
Model Validation: The model’s performance was validated to ensure accuracy in predictions.
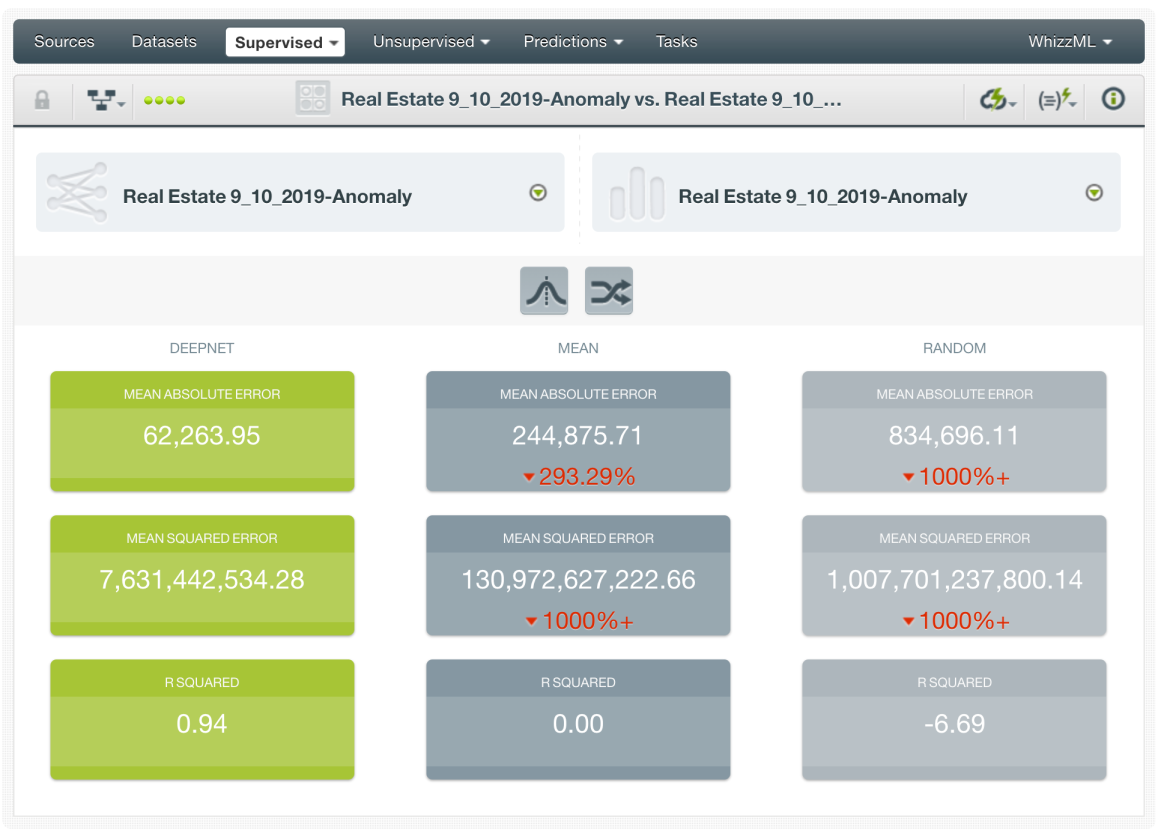
Model validation
Importance fields: here are the predictors
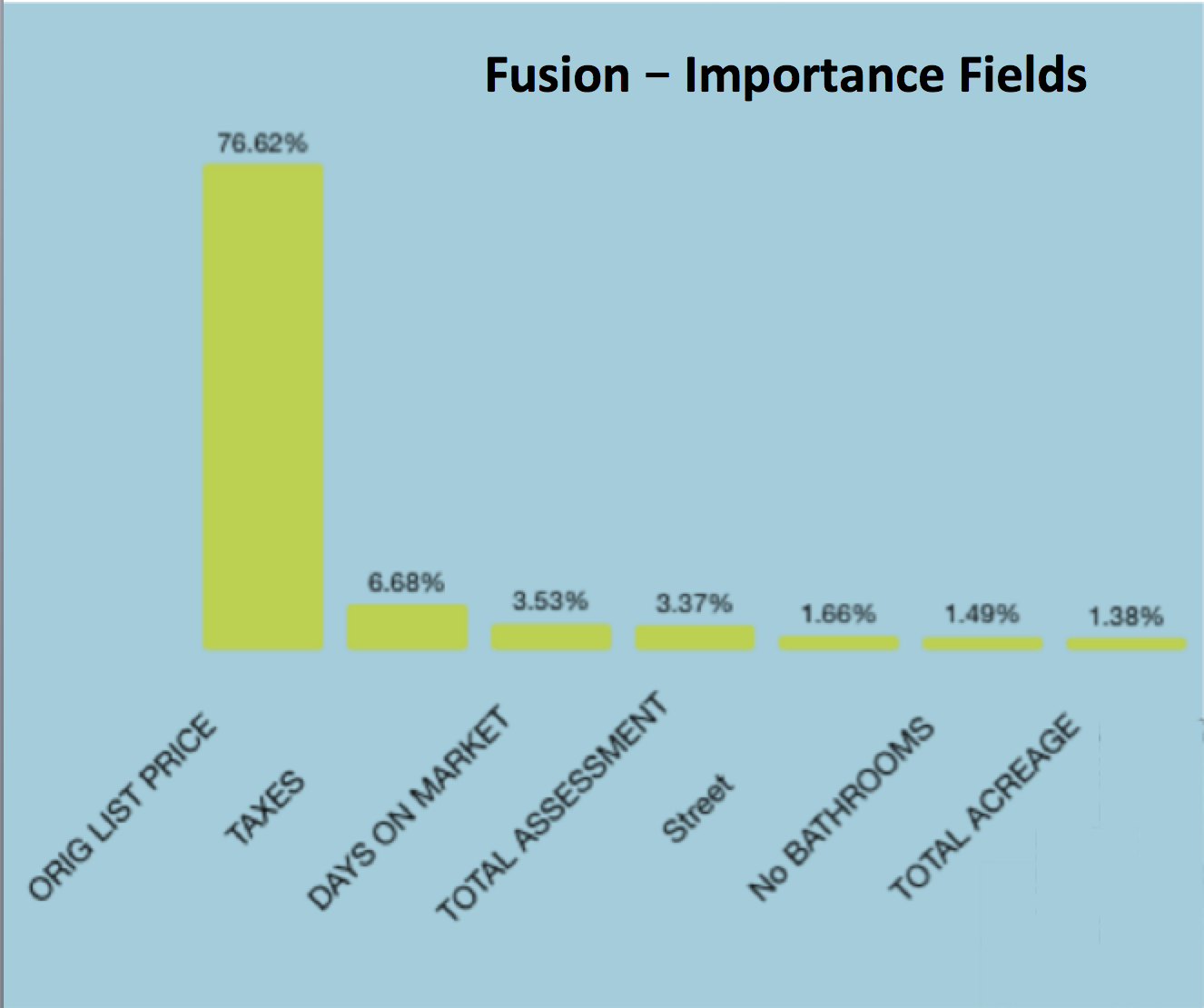
Importance fields
Prediction: Finally, the model was used to predict the bid price for the target house.
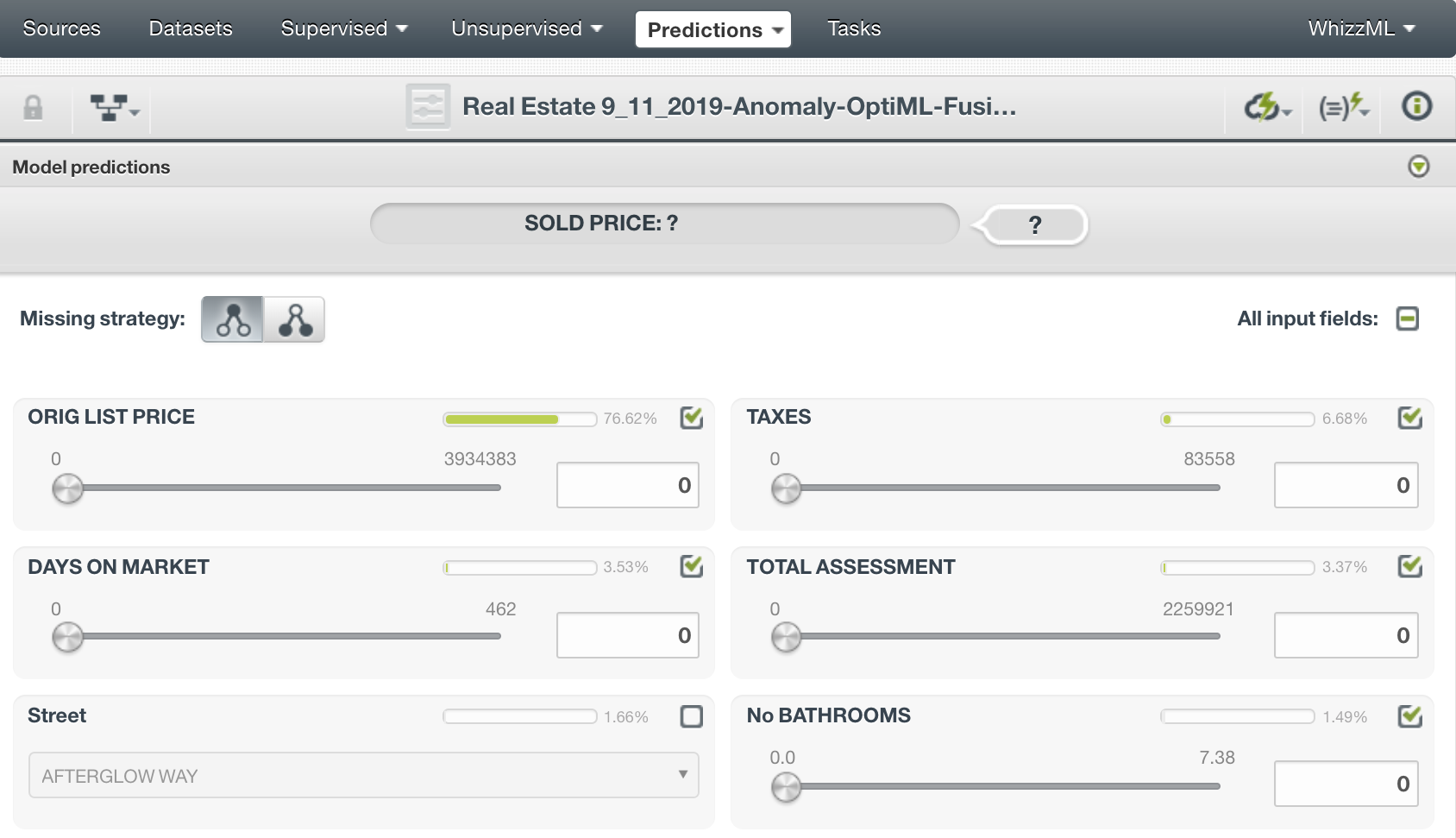
Prediction
Outcome
The predictive model suggested a bid price of $820,587 for the house in question. When compared to the actual sale price of $801,000, the model’s prediction was within an acceptable range, demonstrating its effectiveness in estimating a competitive bid price.

Outcome
Conclusions
Machine learning techniques discover patterns and trends in vast amounts of data, making them a powerful tool for various fields, including real estate. Therefore, machine learning offers a powerful tool for making predictions and informed decisions. As a result, this approach not only helps in making competitive bids but also reduces the uncertainty and stress associated with the bidding process
About me
Francisco Rodríguez is the Founder and CEO of Beanstalk Insight, specializing in marketing research and analytics. He supports small to medium-sized businesses with effective, data-driven growth strategies. Contact Francisco at francisco@beanstalkinsight.com.